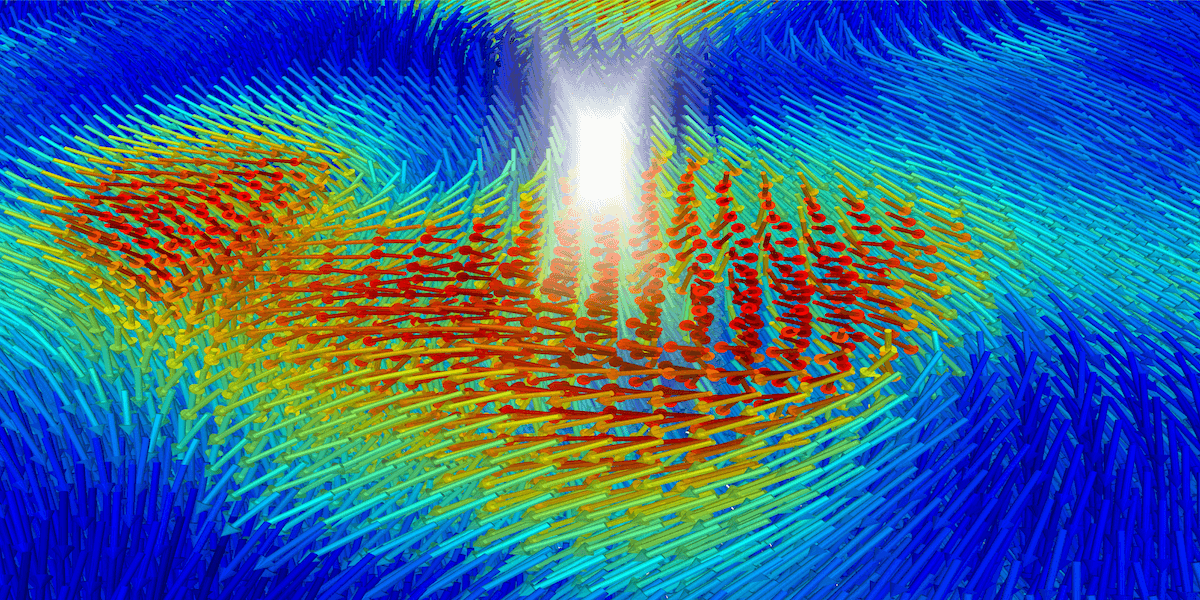
USC Viterbi researchers have created a new machine learning model to investigate how light polarizes materials like lead titanate into a vortex-shaped polarization pattern that vastly improves the material’s energy-efficient properties.
Since prehistory, humans have experimented with changing the properties of materials to make them more useful and desirable; using techniques such heating and stretching materials to convert them into a more practical form — such as melting and then rapidly cooling quartz to turn it into glass.
As science advanced, scientists investigated how to harness electricity, magnetic fields and even light to control a material’s properties. In modern electronics, using light to control materials offers exciting prospects for the development of next generation devices that are more energy efficient and have better performance. Because light interacts with the material on extremely fast time scales, it can uncover properties of the material that heating or stretching cannot. These properties are often referred to as hidden “quantum phases” due to the light interacting with the material at a quantum-mechanical, or atomic, level. However, this makes it extremely difficult to model, which hampers our ability to search for and design next-generation materials.
Materials researchers at the USC Viterbi School of Engineering have developed a new machine learning framework to study at an unprecedented scale how light can control materials. Typical simulations to understand light control of materials can usually simulate only a few hundred atoms, even with state-of-the-art computational resources, which seriously limits their applications. By harnessing the power of machine learning, USC Viterbi researchers were able perform simulations of light control of materials with over a billion atoms — 10 million times greater than conventional methods.
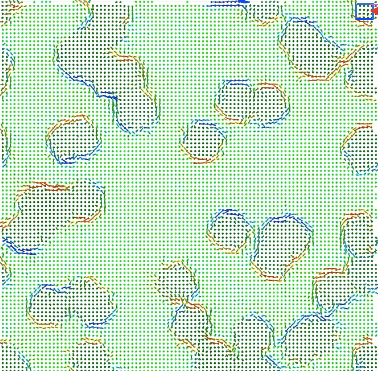
Researcher Thomas Linker said the small blue box is the system size that is currently able to be simulated using standard quantum simulation methods, whereas the entire box is about the scale of what can be simulated with the research team’s neural network.
The research team used their machine learning model to perform large-scale simulation of light control of lead titanate, a special class of material, called a ferroelectric material, that has an inherent electronic polarization. The polarization can be thought of as a pattern of arrows in the material that can be controlled by stretching, heating and electricity, which makes the material ideal for use in sensors, energy storage, and memory. The research, from Senior Lecturer of Chemical Engineering and Materials Science Ken-ichi Nomura, Professor in Chemical Engineering and Materials Science Priya Vashishta, Professor of Computer Science Aiichiro Nakano, Professor of Physics Rajiv Kalia, and Ph.D. student Thomas Linker and their co-authors was recently published in Science Advances.
The researchers examined how the electronic polarization of the material lead-titanate would respond to light. Recently this material has been gaining popularity because it allows researchers to create complex vortex-like patterns in its electronic polarization. When we think of a vortex, we may imagine a chaotic, swirling mass of matter or energy; however, these types of structures have been found to be very stable and efficient in these materials, which is why they are currently being investigated for next-generation energy storage and memory applications. The USC Viterbi researchers wanted to understand if these complex patterns could be controlled with light.
“We wanted to view these large-scale structures with highly accurate simulation methods that use things like quantum mechanics,” Linker said. “But that’s really difficult and very expensive, so we developed a multi-scaled framework where we train a machine learning model to learn a simpler representation of the light matter interaction. Thus, we can simulate much faster.”
“Without machine learning, it would have been impossible to design this kind of simulation,” Nomura said. “By training the machine learning model to learn how the material behaves in response to a strong laser, we can perform our simulation on supercomputers.”
With their framework, the researchers found a new type of phase that was induced by light matter interaction in lead titanate. “If we shine laser (light), we can create a string pattern in the polarization that is topologically different than the original vortex pattern,” said Nomura.
Overall, the research team said their machine learning framework offers an exciting new avenue for exploring light control of materials that was not previously possible.
Machine learning is increasingly becoming a vital tool in the development of novel quantum materials with high performance. The Mork Family Department of Chemical Engineering and Materials Science recently launched a master’s program to train graduates in how to harness machine learning to create advanced materials.
Published on March 28th, 2022
Last updated on May 16th, 2024